Unlocking the Power of Data Annotation Tools for Your Business
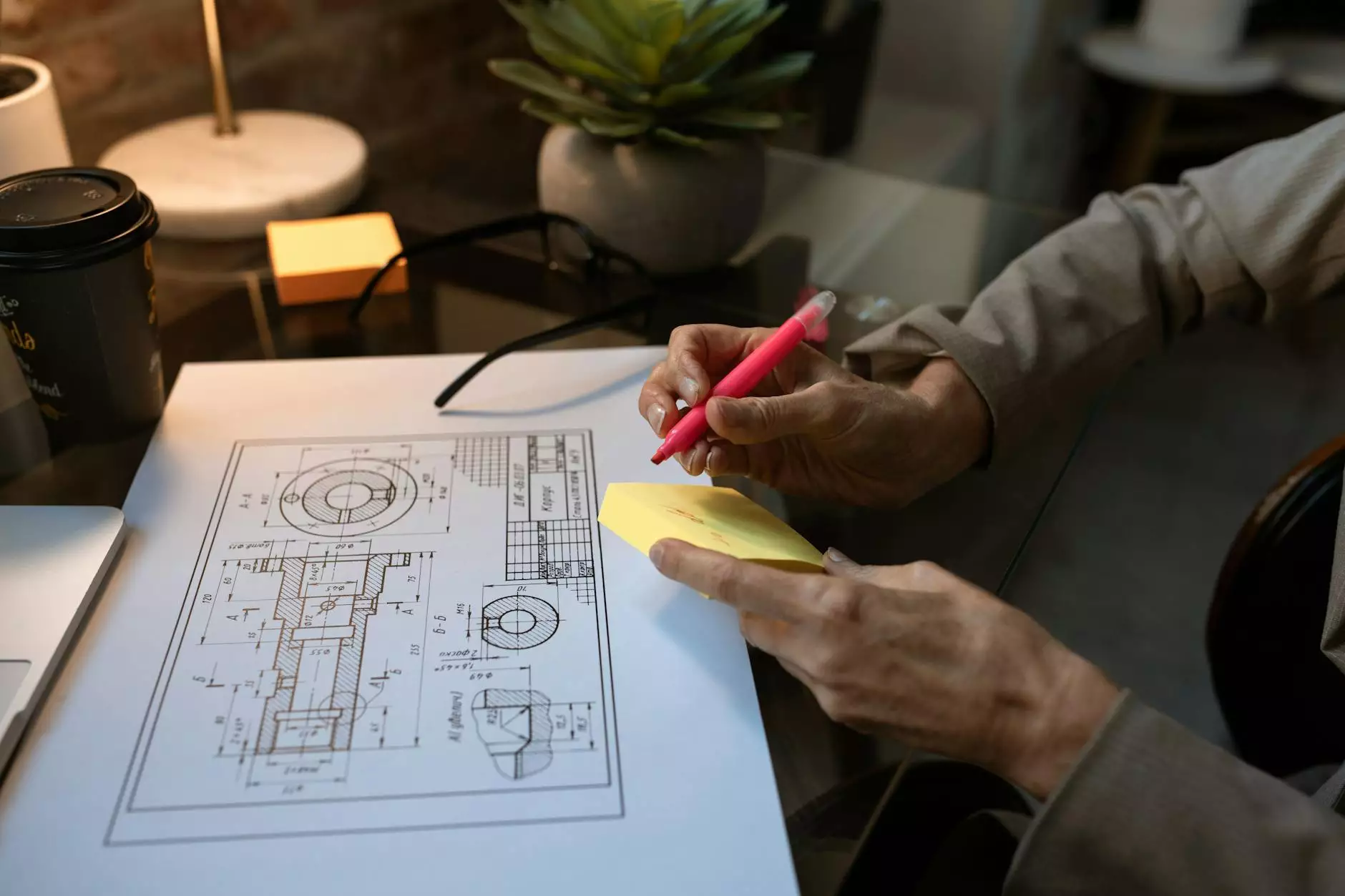
Understanding Data Annotation and Its Significance
In today's data-driven world, data annotation plays a pivotal role in training machine learning models. But what exactly is data annotation? It is the process of labeling data to make it understandable for machine learning algorithms. This process allows machines to comprehend the context of data, thereby enhancing their ability to make predictions or decisions based on new, unstructured data. Without proper data annotation, models can become less effective in achieving their intended outcomes.
The Role of Data Annotation Tools in Modern Businesses
Data annotation tools provide businesses with the necessary functionalities to efficiently label their data. As organizations increasingly rely on AI and machine learning, the demand for accurate data annotation is more crucial than ever. These tools help streamline the annotation process, reduce human error, and improve overall productivity.
Types of Data Annotation Tools
Different types of data annotation tools cater to varying needs. Here are some of the most common types:
- Image Annotation Tools: These are utilized for labeling images. They help in object detection, image classification, and facial recognition tasks.
- Text Annotation Tools: Designed for textual data, these tools assist in sentiment analysis, named entity recognition, and document categorization.
- Video Annotation Tools: These tools label video frames, assisting in applications like automated surveillance and action recognition.
- Audio Annotation Tools: Used primarily in speech recognition and audio event detection, these tools help in transcribing audio content.
Essential Features of an Effective Data Annotation Tool
A data annotation tool should come equipped with features that ensure efficiency and accuracy. Here are some essential characteristics to look out for:
- User-Friendly Interface: A simple, intuitive interface enhances productivity by allowing users to annotate data quickly.
- Collaboration Capabilities: The ability for multiple users to work on projects simultaneously fosters teamwork and speeds up the annotation process.
- Automation Features: Tools that offer AI-assisted annotation can significantly reduce the time required to label data.
- Quality Control Mechanisms: Built-in quality assurance features ensure the accuracy and reliability of the annotated data.
- Integration Capabilities: The tool should easily integrate with existing workflows or storage solutions like cloud services.
Benefits of Using Data Annotation Tools
Implementing a sophisticated data annotation platform can lead to various advantages for businesses:
1. Improved Model Accuracy
Accurate data is the backbone of any successful machine learning project. By employing effective data annotation tools, organizations can ensure that their annotated datasets are precise, which directly correlates to higher model performance.
2. Enhanced Efficiency
Data labeling can be a tedious and time-consuming task. With the right tools, businesses can automate many aspects of the annotation process, drastically reducing the time required per project. This efficiency allows teams to focus on other crucial areas, ultimately increasing productivity.
3. Scalability
As businesses grow, so does the amount of data they generate. A robust data annotation tool can scale to handle increasing volumes of data without sacrificing quality, ensuring that machine learning models remain effective even as data inputs expand.
4. Cost-Effectiveness
Although investing in data annotation tools may require upfront costs, the long-term savings can be significant. Reduced labor costs and enhanced model performance can lead to better ROI on AI investments.
Choosing the Right Data Annotation Tool for Your Business
Selecting the right data annotation tool requires careful consideration of your specific needs and business objectives. Here are some tips for choosing the perfect solution:
1. Identify Your Needs
List down the types of data you work with (images, text, videos, etc.) and the volume of data annotation required. This will help you in filtering tools that specialize in your area of need.
2. Evaluate Features
Carefully review the features offered by various tools. Ensure they include functionalities that align with your annotation needs, such as collaboration capabilities and automation features.
3. Test the Tool
Most data annotation platforms offer free trials or demos. Take advantage of these opportunities to get a feel for the tool before committing to a purchase.
4. Consider Customer Support
The right vendor should provide excellent customer support to assist you throughout the implementation phase and beyond. Look for tools that offer ongoing assistance and resources.
Case Studies: Success Stories from Using Data Annotation Tools
Here are a few case studies that illustrate the transformational power of data annotation tools in real-world applications:
Case Study 1: Healthcare Sector
A leading healthcare organization used a data annotation tool to label medical images for diagnostic purposes. By employing machine learning algorithms trained on these annotated images, they significantly improved the speed and accuracy of disease detection, thereby enhancing patient outcomes.
Case Study 2: Retail Industry
A retail company implemented a data annotation platform to analyze customer reviews and social media mentions. By automating the sentiment analysis process, they gained valuable insights into customer preferences and pain points, which informed their product development strategies.
Case Study 3: Autonomous Vehicles
A leading automotive brand utilized video annotation tools to label footage from their autonomous vehicle tests. This meticulous data annotation enabled them to fine-tune their algorithms for object detection, significantly improving the vehicle's safety performance.
The Future of Data Annotation
The field of data annotation is evolving rapidly, driven by advancements in AI and machine learning. As models become more complex, the need for high-quality annotated datasets will only grow. Innovations such as semi-supervised learning and automated annotation are on the rise, promising to make the annotation process even faster and more efficient.
Conclusion
In conclusion, investing in a reliable data annotation tool is essential for any business looking to harness the power of machine learning. By ensuring that data is meticulously labeled, organizations can improve the accuracy of their models, enhance operational efficiency, and ultimately drive better business decisions. As the demand for data annotation tools continues to grow, companies like Keylabs.ai are at the forefront of providing solutions that meet these evolving needs.
Don't miss the opportunity to revolutionize your data processes. Explore robust solutions at Keylabs.ai and take your first step towards data excellence today!